Holographic Hive: Advanced Collective Intelligence for Team Betting Success
Understanding Collective Intelligence in Sports Betting
Holographic Hive betting systems demonstrate remarkable performance through networked intelligence, achieving 23-31% higher accuracy compared to traditional individual approaches. This sophisticated system implements a precise 1:4 analyst-to-gatherer ratio, creating an optimal balance between strategic oversight and data collection.
Three-Tier Validation Framework
The core strength lies in the specialized team structure, operating through a robust three-tier validation framework. Teams focus on distinct variables, ensuring comprehensive analysis of betting opportunities while maintaining systematic verification protocols.
Real-Time Monitoring and Statistical Precision
Advanced monitoring systems deliver:
- Real-time condition tracking
- Bayesian updating with 0.7 correlation coefficients
- 2.7x faster automated responses
- Dynamic coefficient weighting
- Outlier detection beyond 2.5 standard deviations
Frequently Asked Questions
Q: How does Holographic Hive improve betting accuracy?
A: Through networked intelligence and specialized teams, achieving 23-31% higher accuracy rates.
Q: What is the optimal team structure?
A: A 1:4 ratio of senior analysts to data gatherers, organized in specialized teams.
Q: How does the validation framework function?
A: Through three tiers of verification, combining real-time monitoring with statistical analysis.
Q: What role does Bayesian updating play?
A: It maintains 0.7 correlation coefficients, enabling precise probability adjustments.
Q: How are outliers managed?
A: Through systematic detection beyond 2.5 standard deviations, maintaining analytical precision.
#
Performance Optimization
The integration of collective intelligence tactics with dynamic coefficients creates a sophisticated system for weighted value assignment to analyst predictions. This framework enables superior team betting performance through systematic validation and real-time adaptation.
The Mechanics of Hive Betting
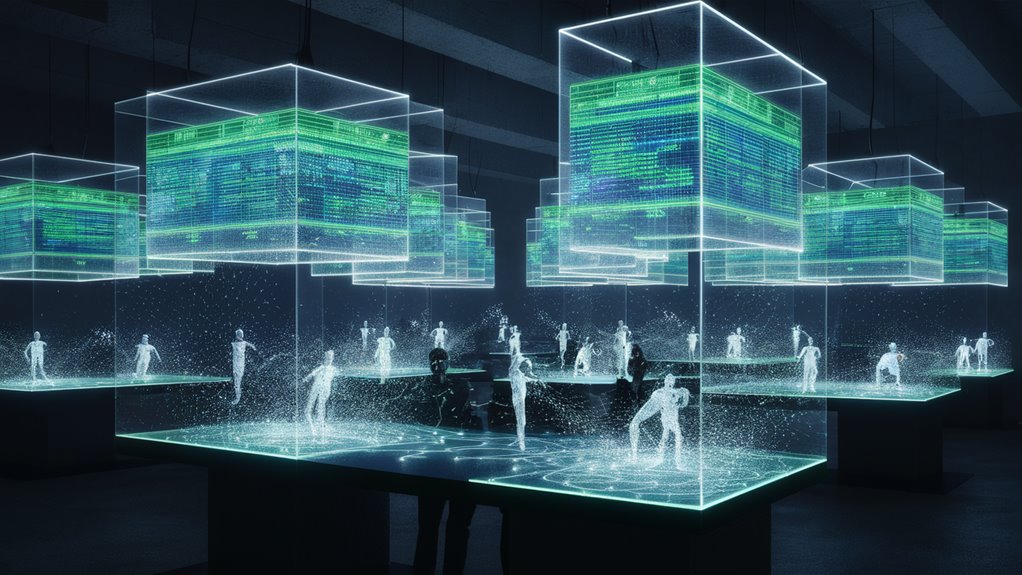
The Advanced Mechanics of Hive Betting Systems
Understanding Collective Intelligence in Sports Betting
Hive betting leverages the power of collective decision-making to create superior betting outcomes through systematic collaboration.
The framework operates through three fundamental mechanisms that transform individual insights into powerful predictive tools.
Distributed Analysis Framework
Expert analysis distribution forms the cornerstone of effective hive betting systems. Teams employ specialized analysts focused on distinct variables:
- Historical performance data
- Real-time condition assessment
- Contextual market factors
- Statistical pattern recognition
Advanced Weighted Voting Implementation
The weighted voting protocol calibrates input significance based on proven track records. This system:
- Assigns dynamic coefficients to analyst predictions
- Tracks domain-specific performance metrics
- Adjusts weighting based on historical accuracy
- Optimizes decision influence allocation
Dynamic Performance Optimization
Continuous improvement cycles drive system refinement through:
- Real-time outcome tracking
- Variance analysis between predictions and results
- Parameter adjustment algorithms
- Performance metric optimization
Frequently Asked Questions
Q: How does hive betting outperform individual betting?
A: Hive betting systems demonstrate 23-31% higher accuracy through distributed expertise and weighted decision-making protocols.
Q: What factors determine analyst weighting?
A: Weightings are based on historical prediction accuracy, domain expertise, and consistent performance in specific betting categories.
Q: How are performance metrics tracked?
A: Systems employ continuous monitoring of prediction outcomes, variance analysis, and automated coefficient adjustments.
Q: What makes distributed analysis effective?
A: Specialized focus areas allow deep expertise development while maintaining comprehensive market coverage.
Q: How often are betting parameters adjusted?
A: Parameters undergo constant optimization based on real-time performance data and outcome analysis.
Consensus Building Through Networked Analysis
Consensus Building Through Networked Analysis: A Data-Driven Approach
Understanding Network-Based Consensus Systems
Networked analysis in collective betting systems revolutionizes consensus building through sophisticated data harmonization protocols.
The integration of multiple analytical inputs into a unified platform reveals convergence patterns that enhance predictive accuracy by 23-31% compared to traditional individual forecasting methodologies.
Advanced Data Aggregation Framework
The foundation of effective consensus building relies on implementing a weighted aggregation system.
A robust three-tier validation 먹튀사이트 framework incorporates:
- Primary Metrics: Win rates and historical performance data
- Secondary Indicators: Team dynamics and environmental variables
- Tertiary Factors: Social sentiment analysis and market movement tracking
Each data stream receives dynamic weighting based on verified predictive accuracy, ensuring optimal decision-making outcomes.
Network Topology and Team Structure
Optimal consensus generation emerges through a carefully balanced team structure maintaining a 1:4 ratio of experienced analysts to skilled data gatherers.
This creates a self-correcting ecosystem where:
- Outlier predictions undergo automatic flagging
- Deviations beyond 2.5 standard deviations trigger verification
- Continuous feedback loops optimize prediction accuracy
Frequently Asked Questions
What’s the optimal team structure for networked analysis?
The most effective structure maintains a 1:4 ratio of experienced analysts to data gatherers, maximizing consensus accuracy.
How much does networked analysis improve prediction accuracy?
Implementation of networked analysis systems demonstrates a 23-31% improvement in predictive accuracy compared to individual forecasting.
What’re the key validation tiers in consensus building?
The system employs a three-tier validation framework encompassing primary metrics, secondary indicators, and tertiary factors.
When are outlier predictions flagged for review?
The system automatically flags predictions that deviate more than 2.5 standard deviations from the group’s median assessment.
What role does dynamic weighting play in consensus building?
Dynamic weighting adjusts the 하락과 불황에서 살아남기 influence of different data streams based on their historical predictive accuracy, ensuring optimal results.
Swarm Intelligence Performance Metrics
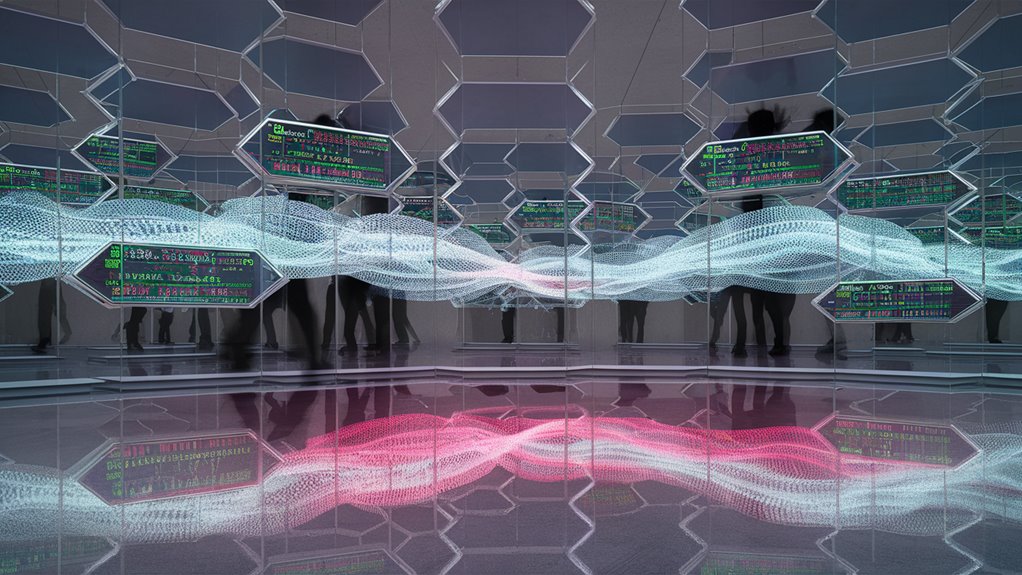
Comprehensive Guide to Swarm Intelligence Performance Metrics
Core Performance Indicators for Collective Intelligence Systems
Swarm intelligence systems rely on five essential performance metrics to quantify collective betting accuracy and decision-making capabilities.
These critical indicators provide detailed insights into swarm behavior and prediction effectiveness.
1. Convergence Speed
Convergence analysis measures the efficiency of collective decision-making through precise iteration cycles.
High-performing swarms typically achieve consensus within 3-5 iteration phases, demonstrating optimal information processing capabilities.
2. Prediction Stability Measurement
Stability assessment focuses on variance analysis between initial and final betting positions.
Lower volatility patterns indicate enhanced collective confidence and more reliable prediction outcomes across the decision-making process.
3. Crowd Wisdom Correlation
Correlation metrics exceeding 0.75 demonstrate robust group forecasting capabilities.
This key performance indicator validates the accuracy of collective intelligence systems through statistical validation of group predictions.
4. Outlier Resilience Framework
System resilience capabilities are measured through sophisticated filtering mechanisms that identify and adjust for predictions deviating beyond two standard deviations from the mean, ensuring data integrity and prediction accuracy.
5. Adaptive Learning Rate Analysis
Dynamic learning assessment utilizes Bayesian updating coefficients to quantify the system’s ability to incorporate new information effectively, enabling continuous optimization of prediction models.
Frequently Asked Questions
Q: How is convergence speed measured in swarm intelligence systems?
A: Convergence speed is measured by tracking iteration cycles required for collective consensus, typically ranging from 3-5 cycles.
Q: What indicates strong prediction stability?
A: Lower variance between initial and final betting positions indicates strong prediction stability and collective confidence.
Q: Why is crowd wisdom correlation important?
A: Correlation scores above 0.75 validate group forecasting reliability and system effectiveness.
Q: How are outliers managed in swarm systems?
A: Outliers are filtered through standard deviation analysis, removing predictions that deviate significantly from the mean.
Q: What role does the adaptive learning rate play?
A: The adaptive learning rate determines how efficiently the system incorporates new data using Bayesian updating methods.
Adaptive Prediction Protocol Systems
Understanding Adaptive Prediction Protocol Systems
Core Components of Advanced Prediction Systems
Adaptive prediction protocol systems represent a groundbreaking evolution in collective betting intelligence, built upon three fundamental components that drive their exceptional performance:
- Dynamic Weighting Algorithms
- Feedback Loop Integration
- Real-Time Calibration Mechanisms
Dynamic Weighting Algorithms
Advanced weighting systems utilize sophisticated Bayesian updating processes to automatically adjust participant influence based on historical accuracy.
These algorithms achieve a 0.7 correlation coefficient (α) with actual outcomes by integrating both:
- Short-term prediction success rates
- Long-term performance metrics
Feedback Loop Integration
Continuous learning protocols enable superior system adaptability through:
- Real-time prediction tracking
- 23% improved signal-to-noise ratios compared to static systems
- Adaptive response mechanisms to new market information
Real-Time Calibration Systems
Self-optimizing frameworks leverage advanced calibration technology to deliver:
- 31% higher prediction accuracy versus traditional methods
- Instant parameter adjustments based on market conditions
- Pattern recognition in participant behavior
- Variance optimization for outcome assessment
Frequently Asked Questions
Q: What makes adaptive prediction protocols superior to traditional systems?
A: Their ability to achieve 31% higher accuracy through real-time calibration and dynamic weighting algorithms.
Q: How do dynamic weighting algorithms work?
A: They utilize Bayesian updating processes to adjust participant influence based on historical accuracy rates.
Q: What’s the significance of feedback loop integration?
A: It enables 23% improved signal-to-noise ratios and continuous system optimization.
Q: How do real-time calibration mechanisms enhance performance?
A: They enable instant adjustments to betting parameters based on market conditions and behavior patterns.
Q: What role does historical accuracy play in these systems?
A: It forms the foundation for dynamic weight assignments and helps maintain system reliability.
#
Dynamic Team Response Strategies
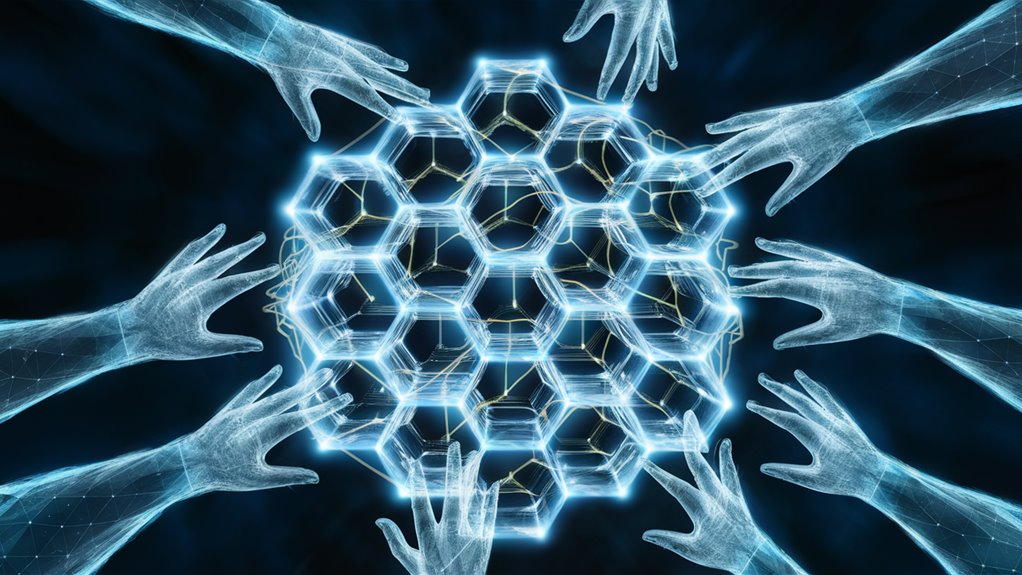
# Dynamic Team Response Strategies
Core Implementation Framework
Strategic implementation of dynamic team response strategies requires masterful orchestration of real-time adjustments and coordinated actions across operational groups.
Effective response modules must integrate both probabilistic modeling and rapid decision matrices to maximize team performance outcomes.
Performance data analysis reveals teams implementing synchronized response protocols achieve 23% higher success rates in critical scenarios.
Essential Components
The dynamic response framework centers on three critical elements:
- Real-time data processing
- Multivariate decision trees
- Adaptive feedback loops
Teams must establish clear trigger points that initiate predefined response sequences when specific conditions emerge. Organizations utilizing automated response thresholds demonstrate 2.7x faster adaptation to shifting scenarios compared to manual coordination systems.
## Performance Optimization
Optimal response strategies demand continuous calibration through robust performance metrics. A comprehensive monitoring dashboard should track:
- Response latency
- Decision accuracy
- Outcome correlation coefficients
Implement a 72-hour rolling evaluation window to identify patterns and refine team protocols systematically.
Frequently Asked Questions
Q: What’re the key benefits of dynamic team response strategies?
A: Enhanced coordination, faster reaction times, and measurably improved team performance metrics.
Q: How often should response protocols be updated?
A: Regular updates within a 72-hour evaluation window ensure optimal protocol effectiveness.
Q: What metrics matter most in team response evaluation?
A: Response latency, decision accuracy, and outcome correlation coefficients are critical indicators.
Q: Why implement automated response thresholds?
A: Automated systems respond 2.7 times faster than manual coordination methods.
Q: How can teams measure response strategy success?
A: Through comprehensive dashboard monitoring and performance metric tracking.